Big Data is a term used to refer to datasets that are extremely large and complex, which cannot be processed using traditional methods and tools. The definition of Big Data encompasses not only volume but also the velocity, variety, and veracity of the data. Volume refers to the massive amounts of data generated daily from various sources such as social media, IoT sensors, online transactions, and more. Velocity pertains to the speed at which data is collected and processed in real-time or near real-time. Variety refers to the different forms of data, ranging from structured data like spreadsheets to unstructured data such as images, videos, and text.
In the context of modern business, Big Data plays an incredibly important role. Today’s enterprises not only stop at collecting data but also need to know how to analyze and use it effectively. Big Data helps businesses gain deeper insights into the market, predict trends, enhance customer experience, and optimize operational processes. This not only drives revenue growth but also reduces costs, increases customer satisfaction, and creates sustainable competitive advantages.
Leveraging Big Data is no longer optional but has become a crucial factor for sustainable development in the digital age. Leading companies worldwide are heavily investing in technology infrastructure and human resources to maximize the potential of Big Data. Businesses that fail to keep up with this trend risk falling behind and losing their competitive edge in the market. Therefore, awareness and effective implementation of Big Data strategies are key for enterprises to achieve success in an ever-changing modern business environment.
Exploring Big Data Performance
1. What is Big Data Performance?
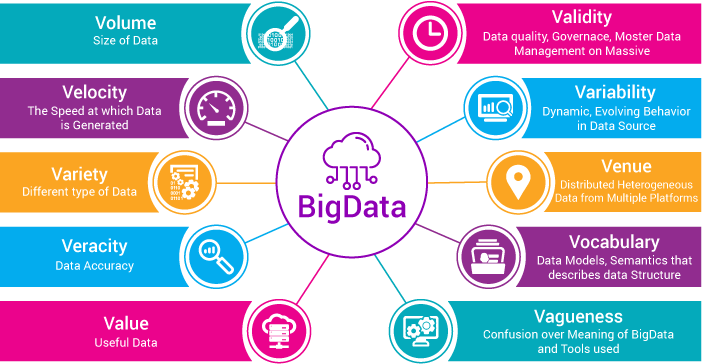
What is Big Data Performance? (Collected)
Big Data performance refers to the efficiency and effectiveness with which large datasets are processed and analyzed to derive meaningful insights. This concept encompasses the ability to handle vast amounts of data swiftly, accurately, and reliably, ensuring that the information extracted is useful for decision-making and strategic planning. High performance in Big Data implies optimized data processing pipelines, robust analytical capabilities, and the capacity to manage and interpret complex data structures without compromising on speed or accuracy.
2. Factors Affecting Big Data Performance
Several factors influence the performance of Big Data systems, each playing a crucial role in ensuring data is processed and analyzed efficiently:
2.1. Volume of Data
The sheer volume of data is a primary factor affecting Big Data performance. As businesses collect increasingly large datasets from various sources, the ability to store, manage, and retrieve this data efficiently becomes critical. Systems need to scale horizontally to handle petabytes or even exabytes of data without degradation in performance.
2.2. Processing Speed
Processing Speed (Collected)
Processing speed, or velocity, is another vital factor. It refers to the rate at which data is ingested, processed, and analyzed. In many applications, especially those requiring real-time insights such as financial trading or fraud detection, high-speed data processing is essential. Technologies like in-memory computing and distributed processing frameworks, such as Apache Spark, are employed to enhance processing speeds.
2.3. Variety of Data
Big Data comes in various forms, including structured, semi-structured, and unstructured data. Structured data, like databases and spreadsheets, is easier to process, whereas unstructured data, such as social media posts, videos, and sensor data, requires more sophisticated analytical tools and techniques. The ability to efficiently handle and integrate these diverse data types significantly impacts overall Big Data performance.
2.4. Data Accuracy and Quality
The accuracy and quality of data are paramount for reliable Big Data performance. High-quality data is clean, accurate, and consistent, which ensures that the insights derived are trustworthy and actionable. Poor data quality can lead to incorrect conclusions and flawed decision-making. Implementing robust data governance practices, including data cleansing and validation processes, is essential to maintain data integrity.
Optimizing Big Data performance involves addressing these factors through advanced technologies and best practices. By focusing on scalable storage solutions, high-speed processing capabilities, versatile data handling, and stringent data quality measures, organizations can maximize the value extracted from their Big Data initiatives. This, in turn, enables more informed decision-making, enhances operational efficiencies, and drives competitive advantage in the marketplace.
Benefits of optimizing big data performance
1. Enhancing Decision-Making Processes
Enhancing Decision-Making Processes (Collected)
Optimizing Big Data performance significantly improves decision-making processes within organizations. Big Data provides detailed, accurate insights that enable quick and precise decision-making. By leveraging advanced analytics and machine learning algorithms, businesses can identify patterns, correlations, and trends within vast datasets. This capability allows decision-makers to move beyond intuition-based strategies and make data-driven decisions that enhance operational efficiency and strategic planning. For instance, predictive analytics can forecast market trends, helping businesses to proactively adjust their strategies and stay ahead of competitors. In essence, optimized Big Data performance transforms raw data into actionable intelligence, thereby enhancing the overall quality and speed of business decisions.
2. Boosting Operational Efficiency
Another critical benefit of optimizing Big Data performance is the enhancement of operational efficiency. By analyzing data from various sources such as production lines, supply chains, and customer interactions, businesses can identify inefficiencies and areas for improvement. For example, real-time data analytics can monitor equipment performance and predict maintenance needs, thereby reducing downtime and extending the lifespan of machinery. Additionally, process optimization algorithms can streamline workflows, minimize waste, and enhance resource allocation. Consequently, businesses can achieve higher productivity levels, reduce operational costs, and improve profitability. In a competitive market, these efficiencies can provide a significant edge over rivals.
3. Personalizing Customer Experiences
Personalizing Customer Experiences (Collected)
Optimizing Big Data performance also allows businesses to personalize customer experiences. By analyzing customer data, such as purchase history, browsing behavior, and social media interactions, companies can gain a deeper understanding of individual preferences and needs. This insight enables the creation of personalized marketing campaigns, product recommendations, and customer service interactions. For instance, recommendation engines powered by Big Data analytics can suggest products that are most likely to appeal to specific customers, thereby increasing conversion rates and customer satisfaction. Moreover, personalized experiences foster customer loyalty and retention, which are crucial for long-term business success. In today’s consumer-centric market, personalization powered by Big Data is a key differentiator.
4. Developing New Products and Services
Finally, optimizing Big Data performance facilitates the development of new products and services. By exploring vast datasets, businesses can uncover emerging needs and market trends that were previously unrecognized. This process of discovery is crucial for innovation and staying relevant in a dynamic market. For example, sentiment analysis of social media data can reveal customer dissatisfaction with existing products, highlighting opportunities for improvement or the creation of new solutions. Additionally, trend analysis can identify shifts in consumer behavior, guiding the development of products that meet future demand. By leveraging Big Data, businesses can accelerate their innovation cycles, reduce time-to-market for new products, and gain a competitive advantage.
In summary, the optimization of Big Data performance offers substantial benefits across various facets of business operations. From enhancing decision-making and boosting operational efficiency to personalizing customer experiences and driving innovation, the strategic use of Big Data is pivotal in achieving sustained business growth and competitive advantage in the modern economy.
Steps to Optimize Big Data Performance
1. Efficient Data Collection and Storage
Efficient Data Collection and Storage (Collected)
Efficient data collection and storage form the foundation of optimizing Big Data performance. To handle the vast and diverse datasets characteristic of Big Data, businesses need to employ appropriate data storage systems such as Hadoop and NoSQL databases. Hadoop, an open-source framework, enables distributed storage and processing of large data sets across clusters of computers using simple programming models. It is highly scalable, allowing enterprises to handle data growth seamlessly. NoSQL databases, on the other hand, provide a flexible schema design and can efficiently store unstructured data, making them ideal for Big Data applications where the data types are varied and evolving. These storage solutions not only ensure that data is readily accessible but also enhance the ability to perform complex data operations without bottlenecks.
2. Data Analysis and Processing
Analyzing and processing data effectively is crucial for extracting actionable insights from Big Data. This involves the application of advanced tools and techniques, such as Machine Learning (ML) and Artificial Intelligence (AI). ML algorithms can identify patterns and relationships within the data that might be impossible to detect using traditional statistical methods. AI enhances this by enabling predictive analytics, which helps businesses forecast future trends and behaviors. Tools like Apache Spark, which supports large-scale data processing and offers in-memory computing capabilities, significantly speed up the analysis process. These technologies facilitate real-time data processing, allowing businesses to make timely and informed decisions based on the latest data insights.
3. Ensuring Data Quality
Ensuring data quality is paramount to deriving accurate and reliable insights from Big Data. Poor quality data can lead to incorrect conclusions and suboptimal decision-making. Therefore, businesses must establish robust data quality assurance processes that include regular data validation, cleansing, and enrichment. Data validation involves checking the accuracy and consistency of data as it is collected. Data cleansing removes or corrects inaccuracies and inconsistencies, ensuring that the data set is free from errors. Data enrichment, on the other hand, involves enhancing the data with additional information to improve its value. Implementing automated tools for these processes can significantly improve efficiency and accuracy, ensuring that the data used for analysis is of the highest quality.
4. Data Security and Protection
Data security and protection are critical in the era of Big Data, where breaches can lead to severe consequences, including financial loss and reputational damage. To safeguard data, businesses must implement comprehensive security measures such as encryption and firewalls. Encryption ensures that data is unreadable to unauthorized users, both during transmission and while stored. Firewalls act as a barrier between trusted internal networks and untrusted external networks, controlling the incoming and outgoing network traffic based on predetermined security rules. Additionally, employing advanced security protocols like SSL/TLS for secure data transmission and implementing multi-factor authentication for data access can further enhance data protection. Regular security audits and compliance with data protection regulations such as GDPR and CCPA are also essential to maintaining data integrity and security.
By focusing on these key areas—efficient data collection and storage, robust data analysis and processing, stringent data quality assurance, and comprehensive data security—businesses can optimize their Big Data performance. This optimization not only maximizes the value derived from data but also ensures that the data infrastructure is resilient, scalable, and secure, positioning businesses to thrive in the data-driven economy.
Tools and Technologies Supporting Optimal Big Data Performance
In the era of Big Data, the tools and technologies we use for data storage, management, analysis, and security are more critical than ever. This article delves into the key components that enable businesses to effectively handle and leverage their data: the robust storage and management capabilities of Hadoop, Spark, and Cassandra; the powerful data analysis tools like Tableau, Power BI, and Google Analytics; and the essential security technologies such as SSL, TLS, and encryption tools.
1. Data Storage and Management Tools
Data Storage and Management Tools (Collected)
In the realm of Big Data, effective data storage and management are critical to handle the vast and complex datasets. Hadoop, Spark, and Cassandra are three prominent tools that have revolutionized this domain. Hadoop is an open-source framework that allows for the distributed processing of large datasets across clusters of computers using simple programming models. It is designed to scale up from a single server to thousands of machines, each offering local computation and storage. Apache Spark is another powerful open-source processing engine designed for speed and ease of use. It enables data parallelism and fault tolerance, making it ideal for large-scale data processing tasks. Unlike Hadoop’s MapReduce, Spark provides in-memory processing capabilities, which significantly boosts performance for certain data-intensive applications. Apache Cassandra is a highly scalable, high-performance distributed database designed to handle large amounts of data across many commodity servers, providing high availability with no single point of failure. It is known for its robust support for clusters spanning multiple data centers, with asynchronous masterless replication allowing low-latency operations for all clients.
2. Data Analytics Tools
For analyzing Big Data, tools like Tableau, Power BI, and Google Analytics are indispensable. Tableau is a powerful data visualization tool used in the business intelligence industry to analyze data and create interactive, shareable dashboards. It connects easily to nearly any data source, be it corporate Data Warehouses, Microsoft Excel, or web-based data, and allows for immediate insight by transforming data into visually appealing, interactive visualizations. Power BI by Microsoft is another leading business analytics service that provides interactive visualizations with self-service business intelligence capabilities, where end users can create reports and dashboards by themselves, without having to depend on any information technology staff or database administrators. Google Analytics, on the other hand, is a web analytics service offered by Google that tracks and reports website traffic. It is a powerful tool for measuring the performance of online marketing campaigns, understanding user interactions, and optimizing websites to improve user experience and increase conversions.
3. Data Security Technologies
Ensuring the security of Big Data is paramount, and technologies like SSL, TLS, and various encryption tools play a vital role. SSL (Secure Sockets Layer) and TLS (Transport Layer Security) are cryptographic protocols designed to provide secure communication over a computer network. They are widely used to secure websites and online transactions, ensuring that data transferred between servers and browsers remains private and integral. Encryption tools are essential for protecting sensitive data. They transform readable data into an encoded format that can only be read or decrypted by someone with the appropriate decryption key. Various encryption techniques, such as symmetric encryption (using a single key for both encryption and decryption) and asymmetric encryption (using a public key for encryption and a private key for decryption), are employed to safeguard data at rest and in transit. These technologies are crucial for maintaining data confidentiality, integrity, and authenticity in the face of increasingly sophisticated cyber threats.
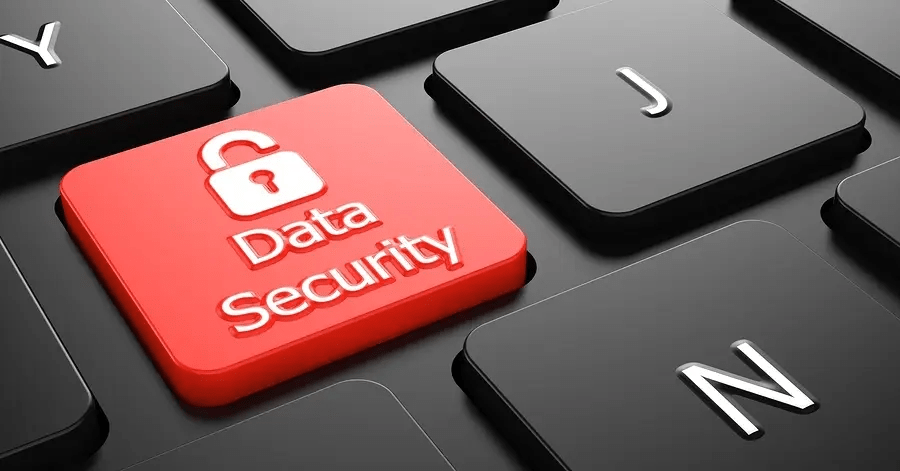
Data Security Technologies (Collected)
Harnessing the power of Big Data requires a comprehensive approach that integrates advanced storage solutions, sophisticated data analytics, and robust security measures. Tools like Hadoop, Spark, and Cassandra provide the necessary infrastructure to manage vast datasets efficiently. Data analytics tools such as Tableau, Power BI, and Google Analytics transform raw data into actionable insights. Finally, security technologies like SSL, TLS, and encryption ensure that data remains protected throughout its lifecycle. Together, these components form the backbone of a successful Big Data strategy, enabling businesses to unlock their data’s full potential while maintaining the highest standards of security and performance.
Challenges and Solutions of Big Data
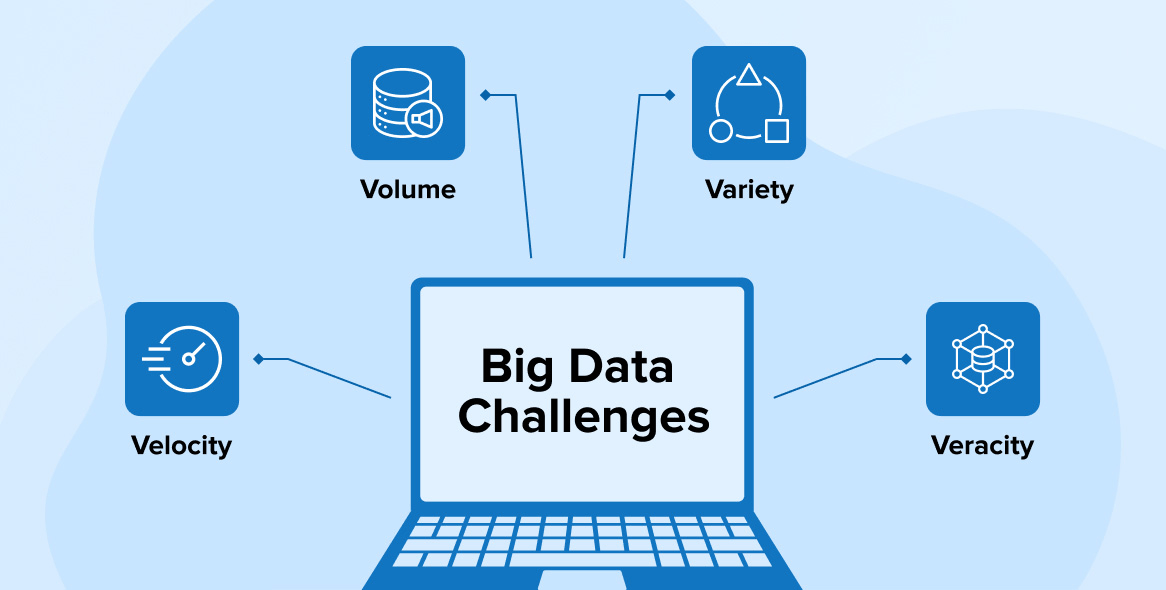
Challenges and Solutions of Big Data (Collected)
1. Handling Large Data Volumes
One of the primary challenges in dealing with Big Data is the sheer volume of data generated daily from various sources. Traditional storage and processing methods may struggle to cope with such massive datasets. To overcome this challenge, organizations are increasingly turning to cloud storage solutions and data segmentation techniques. Cloud storage offers scalability and flexibility, allowing businesses to store and access vast amounts of data without the need for large on-premises infrastructure. Data segmentation involves dividing large datasets into smaller, more manageable chunks, making it easier to store, process, and analyze the data efficiently.
2. Ensuring Data Quality and Reliability
Another significant challenge in working with Big Data is ensuring the quality and reliability of the data. With the vast amount of data being generated, there is a risk of inconsistencies, inaccuracies, and incomplete information. To address this challenge, organizations need to establish robust data cleansing processes and implement regular data quality checks. This involves identifying and correcting errors, removing duplicates, and validating the accuracy of the data. By maintaining high standards of data quality, businesses can ensure that their decisions and insights derived from the data are reliable and trustworthy.
3. Data Security Concerns
Data security is a critical concern when dealing with Big Data, especially considering the sensitive nature of some of the information collected. Unauthorized access, data breaches, and cyberattacks pose significant threats to the confidentiality, integrity, and availability of data. To mitigate these risks, organizations need to invest in comprehensive data security measures. This includes providing ongoing training for employees to raise awareness about cybersecurity best practices and implementing advanced security technologies such as encryption, access controls, and intrusion detection systems. By prioritizing data security, businesses can safeguard their data assets and protect against potential threats.
4. Challenges in Data Analysis
Analyzing Big Data can be challenging due to its complexity and scale. Traditional analytics tools and techniques may not be sufficient to extract meaningful insights from large and diverse datasets. To address this challenge, organizations are leveraging artificial intelligence (AI) and machine learning (ML) technologies to enhance their data analysis capabilities. AI and ML algorithms can automatically identify patterns, trends, and anomalies in the data, enabling businesses to uncover valuable insights more efficiently. By harnessing the power of AI and ML, organizations can improve decision-making, optimize processes, and drive innovation based on data-driven insights.
In conclusion, while Big Data presents numerous challenges, organizations can overcome them by adopting innovative solutions and best practices in data management, quality assurance, security, and analysis. By effectively addressing these challenges, businesses can harness the full potential of Big Data to gain a competitive advantage and drive success in the digital age.
Conclusion
In conclusion, optimizing the performance of Big Data yields numerous benefits for businesses in the modern era. The advantages highlighted throughout this discussion underscore the significance of leveraging Big Data effectively. By harnessing the power of Big Data, enterprises can gain deeper insights into their market, anticipate trends, enhance customer experiences, and streamline operational processes. Moreover, optimizing Big Data leads to revenue growth, cost reduction, increased customer satisfaction, and sustainable competitive advantages.
Therefore, it is imperative for businesses to take action now. I strongly encourage enterprises to initiate the implementation of Big Data optimization steps immediately to realize maximum value. By investing in technology infrastructure, data analytics capabilities, and employee training, businesses can unlock the full potential of Big Data and position themselves for success in today’s dynamic business landscape.
TECH LEAD – Leading technology solution for you!
Hotline: 0372278262
Website: https://www.techlead.vn
Linkedin: https://www.linkedin.com/company/techlead-vn/
Email: [email protected]
Address: 4th Floor, No. 11, Nguyen Xien, Thanh Xuan, Hanoi